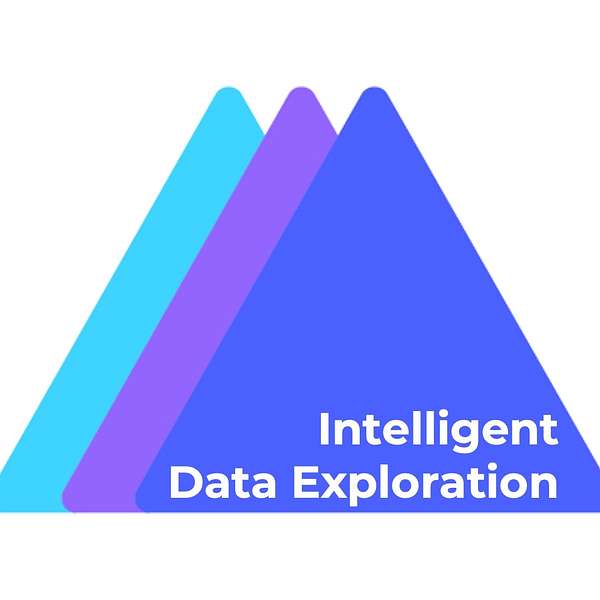
Intelligent Data Exploration
Intelligent Data Exploration
Interview with Justin Smith: Speaking the same language
We're talking to longtime data science leader, Justin Smith, about how the business can work better with the data science teams.
Referenced in this podcast is an AI algorithm to triage patients and known biases in healthcare that impact patient pain treatment.
[00:17] Speaker A: This is intelligent exploration. A virtual Lytics podcast. Hi, welcome to Virtuallytics Intelligence Exploration podcast, where we talk to industry leaders and people doing great things in the space of advanced analytics and AI to pick their brains and learn a little bit more. I'm Caitlin Bigsby, head of product marketing here at virtuallytics today. I'm joined by Justin Smith, a long time practitioner in data science and AI, and a real nerd about AI. And I love every minute of it. Please, I hope you enjoy our discussion. Justin, thank you so much for joining us on the Intelligent Exploration podcast. We're really excited to start doing this series with a bunch of data science professionals talking about kind of anything and everything under the sun. Artificial intelligence is all anybody can talk about it. I feel like I'm seeing it on TikTok of Facebook and LinkedIn because it's just so much at the forefront of our imaginations. But it really is a rich conversation about what it means practically within business and maybe more theoretically. So I thought, first of all, what is your background and what has brought you to the point where you have developed an interest and feel like this is something you can speak about?
[01:34] Speaker B: Yeah, absolutely, no, thank you very much for having me. It's a pleasure to be here today. And it's one of those kind of exciting moments that I enjoy having this conversation to try and move it forward because there's still so much murkiness around. What is AI? What is machine learning, how do we use it, how do we employ it? So a brief background to myself is my bachelor's and master's degrees are in psychology and then I did my PhD in neuroscience. And so wasn't intending originally to get into the world of data or big data or as we were calling it back then, but found myself with some really unique opportunities specifically to start and stand up data science teams. And so it seemed as if a good career fit and this was all pre where we were using the word artificial intelligence like we're using it now, but just a really good overlap in terms of previous skill sets. As a classically trained research scientist, how do you answer these questions? How do you test empirically? How do you use math to prove or disprove what your ideas are? And so it's been a really fun career journey to be able to build, lead and execute with data science teams kind of over my career. And it's impactful in terms of you either come to the same conclusion that you thought you would shown kind of empirically through data, or you come to a different conclusion and say this is where what we believe the facts are and this is how we interpret them. This is the math that we use in being as transparent as possible. And here's the story from it. Right? And so a lot of it, I would say especially from a data science leadership perspective, is the idea of how do you craft that story? Because sometimes you're confirming what somebody's been believing. Usually typically in the C suite or you're showing them that it may be in the opposite way of how they were believing it to be. And so that's always a fun conversation to have. And usually when done well, you set those expectations up ahead of time and you say, hey, we're going to go into this with an open mind. We're going to test these hypotheses. We're going to use math machine learning to come up with and then if necessary or applicable, build a predictive model from this to then help with future state and go forward from there. We'll see kind of where the data takes us in the story, I like to say has an unwritten ending until we get to the ending and then we can start to craft it. And if done well, it's interesting either way. So if it confirms what you thought, good. If it's opposite or different than what you thought, why, how come and what's the difference? That's also very interesting, which is fun.
[04:01] Speaker A: Do you find that people are open to course correction when you have just told them that what they were so sure was true is not great question.
[04:11] Speaker B: So typically what happens is how we set these up, how we set these problems up, or kind of we call them deep insights. How we set them up is that we say this is what we will be using. These are techniques that we'll be using. Do you agree with that? Usually techniques that are like, I don't know what you're talking about. For techniques for machine learning, which algorithms you'd like to use, that's your choice. We say that's our domain expertise, but we say these are the facts we're using. From a data perspective, these data are correct. Do you agree or disagree in not like a lawyerly sense, but sort of getting that yes or no answer? And when they agree and say, yeah, that's what we're using, I believe those data, they are as true as we can confirm them to be. Then when the output or the outset comes out at the output, if it's different, they're like, wow, it's usually a AHA moment. And I love having those. Those are the things I have been chasing my whole life. Right, how does this work? And you have the AHA, wow, now what are we going to do moments. And so those are really engaging. And often by the time you've gotten to that point, like again, you're kind of telling them back, you're reporting back or you're showing them the output, they're on board with you and they're willing at least to say, oh, okay, how do we pivot or how do we change this or that's new? What does that mean for us and how do we adjust?
[05:24] Speaker A: Now that's good to hear because I know. I hear a lot of people say that at the executive level, they'll say on one side, we want to be data driven, and then on the other side, what they really want is the data to just back up what they have already been saying. We're thinking, yes, absolutely.
[05:39] Speaker B: And I think that's a very important part of the initial conversation is to say, look, we are going to this with an apolitical attitude, meaning we're agnostic to the outcome, and we're going to go where the truth takes us, where the data drive us to go. And usually when there's somebody that you can kind of say, like, oh, they really want this answer, I try and be as transparent with them as possible to say, look, I get this is what you want it to go. If you're asking us to test it, that means you're somewhat open to having it be potentially different. How can we have that conversation? The implications are what happens if it's different? And then very few times, but a couple of times in my career, maybe more than a couple, have my heads may say, oh, maybe we should wait to ask that question. I say that's the good idea, because the outcome either entails such a large amount of change or a revisitation of potentially capital expense that's already been spent. Right. And so it's like, we have to see this through, then we can look at how we want to do it next time. And that's okay. I think that's the part, especially when you go to really large organizations, decisions are made on multi year types of cycles, especially big, big decisions. And so that's where data science and machine learning and AI can come in to say, this is the course we're on now. How do we adjust correct is probably too strong of a word, but how do we adjust to what we believe the future state will be? And what is that future state going to be? Right. That's also what data science can help with, too, is what do we know about today and how can we help to use that to predict what's going to happen in the future and then adjust to where we think we're going in the future here?
[07:05] Speaker A: Yeah. And that's really where we've kind of gone. If you look at that Analytic Maturity model from Descriptive Diagnostic, predictive prescriptive, that's the goal, right. To make that jump from what happened and why into what should we be doing instead?
[07:21] Speaker B: Totally. And I think the other fun part is the larger organizations you get into, there's different parts of the organization that different places along that path, and that's okay as well. Right. And so you have one group potentially, that's really sophisticated, that's ready for predictive prescriptive modeling, and you have another group that potentially is just like, I don't even know what I don't know. Can you just show me the basic reports? And you say, yeah, absolutely. That will help bring you along. And so one of the other points I like to make sure I include is the education associated with this is really imperative because we're all getting bombarded, as you said. TikTok has AI discussions on it now, right? So it is hyperly into the common vernacular of everybody that's interested in anything along these lines. And so what it is, what it is not what are the capabilities, what are not the capabilities, those are all really good and I think very important conversations to have upfront to make sure that you're successful, right? Because you have something that's like, you're going to build a generalized data model that will solve all of our problems with one question. It's like, no, that doesn't exist yet. We're not there. Take small bites and make progress, but.
[08:33] Speaker A: That is not where we're at.
[08:34] Speaker B: No.
[08:36] Speaker A: You find your background fascinating, not only because my own educational background is psychology as well. And it's just this incredibly, to me, very versatile degree. But have you found that it helps you bridge that gap between data science and the business?
[08:51] Speaker B: Big time? And I think part of it is coming from a background of both psychology and how I describe it between psychology and neuroscience. So psychology is sort of what's happening externally that you can measure or see typically from a human being. We use animal models as well for research. But how is a human behaving? What's happening, like galvanic skin response, all these kind of things that you can stick something on the outside of somebody typically and measure. Right. Psychology doesn't often get into blood samples, although sometimes they do. But that's sort of when you start to blur the lines, whereas neuroscience is what's happening inside the brain. So what's happening at either a genetic level all the way out to behavior, so you got your genomic expression and protein expression, peptide expression, transmitters, all these endocrine response to your hormonal levels. All these things kind of have this system view approach. And so kind of going back to the idea of psychology and how do you interact with humans, from my perspective, it's the idea of it's a fun conversation to have because you can start to see my background was in stress and decision making for my PhD research. How stressed is this individual and how is that going to affect how this conversation is going to go and how do I make sure I meet them where they are? And the other part, too, is one of the other points I like to make is there's no common language that exists today in any language that we speak of, how to concretely talk about the ideas that we're exploring through machine learning and AI. So we have to get to the whiteboard and we have to draw it out. We have to say, when you say the word risk, what do you mean? Define that for me, because I might be saying the word risk, it means something completely differently. And that's okay. We just have to acknowledge that and step back to do it. And so kind of having that background with the I wouldn't say it was like a human factors focused background, but just the idea of how do humans think and behave? How do humans think and behave under stress, which is when you're making multi million or billion dollar decisions. Those are stressful. Right, true. How do you adjust to that? And then how do you meet them where they are and speak a language that you can both speak and understand each other? And often there's many use cases out there of the individual. The customer requests something to be done from data science team. Data science team doesn't do a good job of engaging up front, disappears for a while, comes back and says, here's your solution and the requester. And the customer is like, what are you talking about? This is not at all what I asked for. And either because they didn't have a clear communication upfront or they didn't say this is what done looks like at the very beginning as well. Right, so that's one of those kind of pitfalls to avoid is agree upon. What does the completion portion look like? And I think that's the idea of is this something like a deep insight that will foundationally shift how you perceive and make decisions in your work area? Or is it a predictive model saying we're going to predict how many customers are going to be in the store over the next two weeks? What does that output look like? So again, those are ideas of from my background, which is fun getting to implore those in Data science world. It's fun. I think it's the best way to describe it.
[11:45] Speaker A: That's good, that's good to find your work fun. Are you seeing so when I've been talking to people, I see a lot more data science has gone from being like a really isolated kind of function, sitting in sometimes it data somewhere and now they're seeing it more in the lines of business. Is that also what you're seeing?
[12:03] Speaker B: Yeah, absolutely. And I think that's kind of going along the track with the proliferation of data. Right. So one of my favorite things to say is we're not collecting any less data, right? We're all carrying around these devices in our pockets that collect audio visual movement, not temperature yet, unless you're wearing something that's like a smart band or something. But we're collecting a massive amount of information in ones and zeros and we're storing it. So now the idea is we want to unlock that potential, right? And there's massive amounts of insights to be gained to help kind of give you that competitive advantage when you have a data science team that's able to engage across the spectrum of your business. And so that's where we are seeing it going from kind of that core functionality of back end business operations. How do you make computers run more efficiently, which is a complete data science problem to help solve, to what types of customers should we be engaging with? When and where and how and when done? Well, it looks like magic when in the end it's really just math.
[13:07] Speaker A: It's worthwhile to do this, it's worthwhile to start embedding data science and data teams within the business. What are some of the challenges that that posed though to having them reporting up to executives who are maybe a little less clear on yeah, absolutely.
[13:22] Speaker B: So the ideas there are to be multidisciplinary is key, right? And so usually when I've seen it be really successful, they'll have say like a business intelligence partner that is an expert in that business line. So if you have solutions, use supply chain as an example. If you have a supply chain executive that wants to have something done, you work literally lock and key with the business intelligence domain expert to say when he says risk, this is what he or she means, right? This is what the executive means, this is what they really are interested even though they're talking about it this way. That's different than how finance may talk about it, but it's the same key core concept. So having the collaboration is very important and then being again very clear on what the outcome looks like. And then the other thing I would say too is making sure that data scientists have it's a team sport, I think is the best way to describe it. Right? It's a team sport in the sense of you can't do it as an individual because either the brevity of the question you're trying to solve is so large that you don't want to make a mistake and say the wrong thing. And unfortunately, we're humans and human error happens. And you can run or build code that appears to be working, is giving you an output. But I think for the wherewithal of the business they need to have the ability to check that. Right. And so typically where it's been most successful is you have data scientists hired in pairs or small teams at different pockets or as a one centralized data science team that has kind of different like the hub and spoke versus the distributed model. Right. So how do you want to think about setting those up? And then the other thing that data scientists must have is support on the back end, meaning the compute power. And that's either you can do it through the cloud or on premises however you want to do it, but most of the data sets now are so large where typically you want to do it off of any sort of laptop or desktop computer. Plus it's specifically designed for that. And then you're even going to be limited in what your compute power can be. So figuring out what servers you're going to use? How does that support it? Who is your database administrator? Dba support? So it's kind of this multifaceted, multi team type of approach. The other thing I love to say is data science. It's like golf you never win, golf you're never done. Okay, we've solved this question. We have this model now running in place and we've got this, you know, we can tell how many customers are going to be in the store in the next two weeks. Now what? Right, okay. Now how do we optimize it again? What kind of customers are they? Right? Do we need to change our staffing based on what's happening with customer foot traffic? Those are all kind of old problems that have been solved, but they're good examples of saying, okay, great, what does that mean and how do we adjust to it now, what's that next kind of question. But yes, so I think the pitfalls are if it's done in isolation, right, if it's done without or very little support. And data scientists, we're highly motivated, we're highly creative, and we love solving problems. And so giving them the opportunities and the support that they need to leverage those skills is paramount. And when you're not doing that, it's really hard. I think it's the best way to describe it. You want to be on an island, that stuff.
[16:30] Speaker A: Yeah, I know that makes a lot of sense. How do you see data scientists pairing with business analysts in the business? How do you see that kind of handoff and transition? Do you see things sort of originating and doing some of the legwork or maybe getting overwhelmed and passing it over? Like, how does that relationship ideally work?
[16:49] Speaker B: Yeah. So where I've seen it be very successful is you find I call them our champions, right? So you find your champions, the people who are super excited about what does this mean, what is machine learning, what is AI, what is data science? Kind of from the business intelligence side, the analyst side. And at the very front, I'm like, hey, look, we're going to give you the deep dive course. We want to show you what we do to the level that you're like, I'm full, I've had enough, but I think I get it now. Right? So giving them that level of transparency that they're asking for, that they're eager to understand, like what is machine learning, what are predictive algorithms? What does deep learning do and how do we solve it? Right? How do we use to solve problems? Giving them that education and then they're out typically meeting with their business line customers or whoever they're engaged with and they can start to hear and they get their wheels turning up like, oh, the customer doesn't know they're asking for a predictive model, but I think they would be very much benefited for it. So often it'll be the business intelligence individual kind of hears. Like there might be interest here, they'll come back and contact the data science team. Typically that's sort of the responsibility of the data science leader to then go and say, hey, we have a couple of ideas. Is this something you're thinking about? And they're like, I am thinking about that. So you look a little bit like a wizard because you're coming to them saying, we have some solutions that might be a good fit for you. Let's work together, let's start something. And then you kind of go through and following whatever process or protocol your system has or your organization has to, how do you stand up projects, right? But getting it going. And that's also where you rope in that business intelligence individual, that analyst, to say, help guide us through this process because you know the customer far better than we do. Data science teams are also very limited in the amount of capacity, typically because there's just not a ton of data scientists walking around and so they're full often with the workload. So it's kind of getting on their radar is key. And that's where I think it's really imperative for as the organization at the really high levels to say what are our focus areas for strategic growth or where are we going to focus our strategy and our efforts? And being really crystal clear on that and making sure that if a request comes in, if you can't align it like not dotted line, but draw a line to this, supports this initiative, then it's probably one of things. Like, this is interesting. We can put it on the docket, but we're not going to get to it until it can align to the things that we know can really drive where the organization is going.
[19:08] Speaker A: That brings the thing interesting to me. I've worked quite a few data scientists over the years and I've observed love what they do. It's fun. It's fun and nerd out and sometimes just pursuing for the sake of pursuing is super satisfying, which is great. I mean, it's good to love your work that way. But in order for the organization to justify the continued investment and adoption because building an AI model is one thing, even if it's the perfect model, you still have to get people to use it. You got to roll it out, get people to use it. So there's a lot of organizational shifts and change. You might have to change the way you work, all that stuff. Making it a business imperative, making a business case for it is really important. Do you think data science teams are good at that or is that something they need more help with?
[19:51] Speaker B: Yeah, I would say then we're starting to kind of get to that blended role which sort of is beginning to emerge now. Typically I would say today we call them like data science leaders or head of data science type of positions where they're able to say, okay, this is what the business is asking for. This is the core team of extremely talented individuals that can write the code, build the models, maintain the algorithms, maintain the outputs. How do we bridge that gap so that's the idea of keeping the rent wrangling is kind of one of the fun terms to use, right, in a very positive way of, like, you were doing this project great, and you saw three other things that you can optimize along the way that were not part of the request. Save those, document those in your notebook, keep a note of that, and potentially we can come back to them. But right now, this is the one we're focusing on and just making sure that you're really kind of and I'd say from the leadership perspective, you're clear and you're checking with your team, making sure you're making progress along. Those fronts because yes, again, these are highly motivated, creative individuals, and they're going to chase things down whether they've been asked to or not on their nights and weekends, because it's really engaging work. Especially when they start to see, like, nobody asked for this. This is a massive ROI right here, and I can solve it. And so that's the part where having that kind of conversation to loop back around to the business to say, look, you asked us this. Here's the solution. We're providing it for you delivering. We've met your ROI, but also we found this. That's where it gets to be really exciting because it's those unknown unknowns that you kind of discover along the way, and then they become known unknowns, and then you can solve those, and then they're known knowns, and you're really moving it a lot further. And the businesses we see do that. They accelerate it at such a rapid pace where the best way to describe it? It feels like cheating. Like, you have the cheat codes because they're able to see and predict and move faster than their competitors. And when they do that, it's like, wow, they're really good. Yeah, they're organized. They have really clear strategic objectives. They have a data science team in place that can meet those, and the data pipeline already in place as well, to be able to feed that fuel to the fire that they need for those insights. That's where it's really kind of I don't know if you could tell. I get excited about it because it's super invigorating.
[22:08] Speaker A: Yeah, you get that data pipeline, you get the apps working, making the predictions, you're golden. But again, how has your team helped the adoption on the other side? Because you still need those lay people to pick it up and run with it.
[22:22] Speaker B: Yeah, absolutely. And that is one of the considerations, I think, that happens internally to the data science team, is how are we going to show this information? So it's completely, ideally, completely intuitive to use meaning as a human beings with a neuroscience background, comes back into place. Right. If you show me a graph, and I don't know anything about graphs, and there's one line that's sticking up above all other lines, as a human, I say that's different. What's that? And so then you get into kind of that idea of the visualization, the use case scenario of where they're going to see this information, how are they going to see it, how is it going to inform the action that we want them to take, the decision we want to help them make? And when done well, it seems like, oh, yeah, of course, I just do it. It's just there. And I can see it now. And I can see that this thing happens when done not well, is to jam a square peg into a round hole and it's like you got to leave this system, log in three times over here, go around the building twice and come back and it might be written in chalk on the ground. Nobody's going to use that because it's not available. So it's layering in those insights in a place and time that the end user or the customer is going to be able to pick up and look at. And again, too, I often say that you want it to be really intuitive so that they're not trying to sit there and think like, do I trust the data? Do I trust the math? Do I trust the algorithm? That's all kind of inherently built in. That's what we work on really hard on the background to say, how do I build trust with you? How do I show you? If you want to see, like, we're happy to sit there and explain mathematical formulas to people. We very rarely get that interest. Or my fun part is we say, absolutely, we sit down. They're like, oh, okay, I'm good. It's a four page problem. I don't need to go through these 60 variables. Like, I'm, I'm, I understand and I trust what you're going for, but, but yeah, that idea of, you know, the change that comes along with it, making it so it's an intuitive change that seems like, oh, yeah, why are we doing this the whole time? That's when it's done really well and adoption is extremely high. And I think there's a lot of use cases for the opposite of where a data science team produces something and it's just either hard to get to or it's not intuitive or it's like, here's a six axis graph. Good luck. Humans don't think in six axes. We think in maybe four dimensions at best. Right. And so meeting us where we are as humans, and that's where I think, again, having that neuroscience background, how does the brain work? Is really helpful.
[24:42] Speaker A: Yeah. How does the brain process information and make sense of it and kind of take it in? Like with you, I have been in analytics for a while, and I still look at. A dashboard with multiple sort of tables. I'm like how's the best way to connect these totally doesn't always work. Yeah, I get that. So your background is in healthcare, and it just remind me one of the I think there was an algorithm rolled out actually at the beginning of COVID that was supposed to help triage patients. And it was eventually found that one of the big drivers that it used was amounts spent, previously spent on health care to determine obviously flawed. I can see there's a long history of bias in healthcare, so I imagine you were using that historical. So I'm just imagining, in case how would you develop a model that would be meant to because there's the potential to accelerate, say, diagnoses or Triaging or whatnot is incredible, because it's just so much more information, but at the same time, it would need to be paired with the human capacity to decide whether to go ahead with it or not.
[25:47] Speaker B: Yes.
[25:48] Speaker A: Have you dealt with that? What are your thoughts?
[25:50] Speaker B: Yeah, absolutely. I want to say it's clear in my mind and so kind of how I think about it is the idea of I'm very specific when I work with individuals, specific in health care, but other industries as well is the idea of any model output should never supersede critical thinking or your expertise and judgment. Right. You are the human being who's been doing this for X amount of years, typically have gone to school for a long, long time to be an expert in the area that you're working on, whether it's engineering, manufacturing, marketing, healthcare, wherever it is. Right. And so the outcome, if you as a human look at it and say, it seems weird, something about that doesn't seem right, that's the moment to hit that pause button to say, what is this outcome telling me and how do I look at it? And typically, when done well and you have a good relationship with your user users or the individuals who are the customers asking you? Usually it's me gets the phone call and say, hey, something's up. Like the last three times I've looked at this, it's been kind of weird, what's going on? And that's a trigger for us then, to go and look and see how the data sources changed. Has there been something that's updated? Are we no longer capturing a field that's being baked into the model? And that's usually what's happened is like, oh, nobody told us because nobody told the fourth person down the chain that we're going to turn this server off and we're no longer going to have this information flowing in. And so that's going to affect but it's usually the human kind of at the end that's going to be the one that's going to see that first. Not all the time, because we have safeguards in place, but it's those really subtle changes that have sometimes large effects. And we definitely work on keeping our eye on all of those things. Best practice to do that, right? But sometimes it happens and you don't know, and then you catch it. The other portion of that is the idea which I think you were alluding to is how we think about it is human in the loop of decision making versus human on the loop of decision making. And so the very subtle difference there is in versus on. So if you're in the loop means that you as a human are doing something. It goes through this process, and you get a response back, either a number or a color that says green, yellow, red, red means you should think or do something with this. But then you have a decision to make as the human. Right. An automated system where the human is on the loop means that the system is just making decisions and doing things, and it's your job to kind of check in with it when you think it's necessary. And where we see specifically in areas that involve risk to humans harm or safety or things where it needs to be monitored closely. And decisions made in the loop is really imperative for those types of models. And I'll use automated driving as a really good example. So we keep saying we're going to have fully autonomous vehicles, but the ones that are commercially available now that you can buy, you're still going to touch the steering wheel every three to 10 seconds, whatever it is. Right. So that's you in the loop, you're still driving a car. But again, knowing how humans operate, we're super lazy, and so we just hold our hand in the wheel, but we're talking to our friend in the passenger seat or the back seat, or we're gazing out the window, not actually paying attention. That's where we see really negative outcomes. And so I think that's the part, too, of making sure everybody is clear and understands between those two concepts of human in the loop or human on the loop. And when it's not consequential to human health or safety, putting human on the loop, that's totally fine. That's very appropriate in certain areas, but it's also very not appropriate in certain areas. And so making sure you're understanding what is the outcome and how it's going to be used, and is there a human that should be responsible for that decision or not. This gets into kind of the whole ethical side, which is fascinating, but where does that line lay, and how do you even recognize that you're close to the line? That's another big conversation to have, too.
[29:32] Speaker A: Yeah, I find that interesting because especially things where there have been historical biases towards disenfranchised groups, AI has the potential to do for bad, where you bake in the bias to the AI and then people act on it unthinkingly. It also has a potential for good if you corrected it the other way to make perfectly new one. For example, African Americans are often thought to be able to experience tolerate higher pain levels, for example. So if your AI doesn't do that and recommends, no, this person needs pain medication now, but the doctor is biased. And then therefore so it's a chance to actually sort of challenge maybe the biases of the person acting it or change things. I was really curious, when is it good to bring the human in to check the AI and when is the AI the one checking the potential to challenge or check the person?
[30:27] Speaker B: Yeah, no, I think those are great concepts. And how I think about it is the idea of AI in its current state of the technology. It's a tool in the toolbox, meaning if you know how to use the hammer, you can use it appropriately and you can be efficient. Right. We just saw with our friends over at OpenAI who released Chat GPT to the world, right. The hammer has gone from being a physical hammer, you have to hammer in your nails to a fully automated robot that you could just say, put this board up and it goes and does all the work for you and how do we use that technology now? Right, so it's a tool in the toolbox and then the idea too is what you start to see is from the human side using that tool, you could say, am I using this appropriately? Am I getting challenged every single time I'm making decision and I'm in opposition to the AI. Typically, I would argue how currency of technology is most likely the AI or the machine learning is not correct. But in that very rare instance, it might be you had somebody that's making their decisions in a way that's outside the norm. And so then that's kind of the idea of if you see somebody ignoring it or sitting, no, I'm not going to do that five out of 1000 times or whatever the threshold is, right, that's normal. If you see somebody that can ignore 98% of the time, you got to go and say what's going on? There's something happening here. Either the machine learning is incorrect. But if you're with a cohort of groups or cohort people that are doing the same tasks or tasks and they're all using it appropriately and then there's the one outlier. I think that requires further investigation to say is it a training compartment and how you were trained to do this thing or the decisions to make person who's making the decisions. Or is there something that you're just not participating like something else is going on? And I think that's where using tools like machine learning and AI, we start to see those, whereas if you're not using them, you would never see that. It just would go undiscovered because it's typically a part of the workflow that may not be highly visible.
[32:28] Speaker A: Yeah, no, I think you're right there. There reminds me of somebody I was talking to yesterday. I was saying that obviously we're going to continue to advance in AI, machine learning and so on and so forth. But what's really been the biggest advancement is our access to it. So all of a sudden all these people feel like, oh my God, it's magic. Magic is here now because they can interact with it and wouldn't previously. They couldn't. But I do think it does really call out sort of that we are very ignorant of how it affects us. You see it in the algorithms on YouTube and so on, and the increased division in the world like the way we do, because the content that now gets fed to people. So if you could say anything to anybody about AI, what they really need to understand, what would you want people to understand about it?
[33:12] Speaker B: Yeah, that's a big question. So I think what I would say is take a moment pause and recognize in your life how often you interact with technology. And for most people that I speak with or interact with, the answer is more or less constantly. And so if you're constantly interact with any type of technology, you are creating data. If you are creating data, that data is being used or leveraged or made an opportunity for either improved services or, or a creation of a different way to interact with you that's more efficient. And so kind of those pop up shortcuts that appear on your phone that you're like, oh, I do use that app all the time at this time of day. Right. So yeah, I do want to click that and put it right here. That is machine learning right there. How often is that happening? And I think the moment that has transpired in the public very recently is like, oh my gosh, look at how incredible this technology is. We're in reality, it's been happening for probably the last six to ten years. Just kind of slowly the background optimizing it so that you don't recognize like, oh, your battery performs 40% longer. It's the same size battery, but we're controlling the software differently based on your use in your mobile device that you now can get a lot longer battery, and we expect that we see it. The example I like to use is when somebody goes in to take a shower in the morning. If you're showering the morning or night, whatever it is, you just turn the water on and you step in, you take your hot shower. You don't care at all about how the water pressure is functioning. You don't worry about where the water is coming from because we're trusting that it's safe, right? We don't worry about the salinity or the chemical composition of what's inside the water. Again, we're taking that safe. We're assuming that all the piping is done. Well, we're not having any major leaks somewhere. So all these things happening in the background and we're just the ones who use it. Kind of what's happened recently and transpired is we all just got to have the experience of like, wow, hot showers are awesome. Let's do that. That's a great use of this technology. And so I think we're at another pivotal moment, I would argue, and I've argued in the past, we're in another pivotal moment where we haven't had something like this happen to us as human beings for probably around 10,000 years. And that is when we went from being nomadic to discovering and using technology in agriculture, using agriculture as a technology. So we can now grow our food. This is us 10,000 years ago, right? Our ancestors, we can now grow our food and we don't have to chase these migrating herds around or follow the berries, the seasons, and use a lot of energy to expenditure there. So what we're using now is the shift. And again, I would use Chat Ept as the current example. If I'm going to write a performance review for somebody, I can put in the bullet points and the ideas and the highlights, hit go and it comes back with a performance review for me that I can then edit and say, yeah, this is exactly what I was going to say anyways. I didn't compose those sentences, but the ideas are there. And so as interacting with technology, as human beings, be aware of how often are you interacting with technology? What is the technology then kind of using your information for? And it sounds very Big Brotherish, but I think it can definitely be done for good as well. Right. So I'm an optimist and how do you leverage that and make sure it's being used appropriately for you? And that's the part where I think we can be better as a society and basically a species as well, of we have this new tool, let's use it for good. I use hammers as my example. Hammers are great for building buildings, but they can also solve conflicts with your neighbors. Let's use it for building buildings and not solving conflicts, resolving conflicts. And that's a different outcome. So I think just being aware of what the technology that you're using and how is it being used is really important and hopefully we'll get better at that. And I think we're starting to see that with the more focus on bias or discussions happening around ethics. Right. Just because we can. Should we? I think that's an important question is the idea of, yeah, we could build that, but should we build that? Is that the most appropriate use of this technology and kind of moving forward as a species that we just haven't ever had anything like this before and how do we leverage it for good, not evil?
[37:20] Speaker A: Great. Yeah. I love that summary. Maybe one more thought. We were talking about interdisciplinary teams again, so maybe what would be your advice for somebody who suddenly finds themselves responsible for data science in their line of.
[37:33] Speaker B: Business who's never yeah, absolutely. I would say sit down with the data science team and just have them give you a very high level overview of what their skills and capabilities are. Don't go into dweeds too far and just say, hey, do you have maybe one or two examples of some of the work that you've done previously? Can you show us that and talk us through how the process worked? Right, so a lot of data science is very process driven. We didn't even get a chance to talk about some of the processes involved and that's okay, but what process do they use? How do you have that common language? How do you establish that common language? And then how do you make sure that when you say X, the other person receives understands you mean X, not Y. It comes through. I think the idea of communication and that's the idea of if you're, if you turn data scientists loose without direction or purpose or kind of that idea of wrangling, they're going to solve problems. It might be what they think is the most important problem with the largest hiring they see, but it might be separate from what the business leader is looking at or what they want to do. And so be able to have kind of that guidance is interesting. And I would say also for the business leader, it's really important for when you're engaging with data scientists to say, look, when you see something and you'd make a discovery, right, write that down and let me know. I want to know what you're going to find because I don't know it. And you're going to see things that we haven't even thought about before because you're going to be the one out in the front lines poking around figuring out, like, there's a cliff here. Who knew that we thought this was a flat prairie forever and now there's a canyon. Like, this is amazing. Come back and tell us, hey, there's a canyon here, how can we leverage that? Right? What does that mean? So it's giving them the purview to be creative as you're really important as well, and just say, look, you're going to see things that we don't know about, tell me about them and I'm interested, I want to explore with you, help us move forward. And that's where data scientists get really we get really excited because that's what we want to do is move things forward.
[39:23] Speaker A: That's great advice. You've said that a couple of times about the vocabulary and the communications. And I think you're spot on there because there's a very deliberate language set used in data science that the business needs to understand and vice versa differently. And you really need to be really clear about that. I had a colleague used to call it following the scent of data. When you pick up the scent, follow it, see where it takes you and note it down, because the possibilities who knows what the possibilities are? And the business, there's a one sort of task to act on it and kind of recognize the potential and spin it out. So I think that's excellent advice, Justin. I think that's probably a good place for us to wrap it up.
[40:05] Speaker B: Wonderful.
[40:06] Speaker A: So thankful that you came and sat down and chatted me. It was super interesting and I really like your perspective and I like knowing with all the directions at a psych degree, absolutely.
[40:18] Speaker B: Who knows, you could get neuroscience and they get the data science.
[40:23] Speaker A: The possibilities are endless.
[40:25] Speaker B: Yeah, it's an open world. I think that's what I like to tell people too, is it's a small world. There's lots of opportunities. You never know where the next opportunity is going to go, but it's always exciting and it's a fun place to be, for sure.
[40:38] Speaker A: I want to thank Justin Smith, data scientist and AI enthusiast, for joining me today. It was really fun conversation. We kept recording after this and it was really, really I could have talked for ages with him and stay tuned. We'll have some more interviews in the coming weeks.